Smart analysis of microbial data treasures
Jörg OvermannProfession:
Microbiologist
Position:
Scientific Director of the Leibniz Institute DSMZ- German Collection of Microorganisms and Cell Cultures in Braunschweig and University Professor of Microbiology at the TU Braunschweig
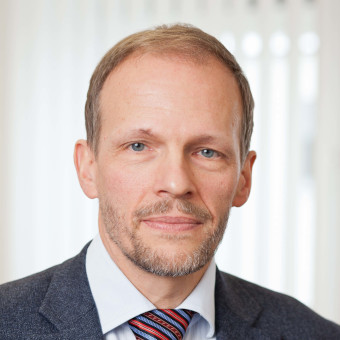
Profession:
Microbiologist
Position:
Scientific Director of the Leibniz Institute DSMZ- German Collection of Microorganisms and Cell Cultures in Braunschweig and University Professor of Microbiology at the TU Braunschweig
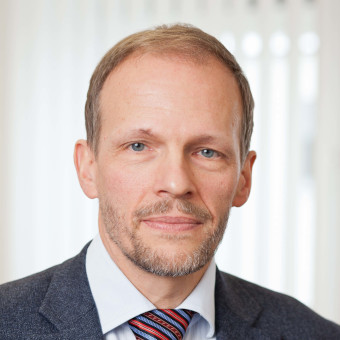
Microbiologist Jörg Overmann wants to investigate the diversity of bacteria and relies on artificial intelligence.
Jörg Overmann is Scientific Director of the Leibniz Institute DSMZ-German Collection of Microorganisms and Cell Cultures GmbH and leads the world's most diverse archive for biological resources. Microorganisms and cell cultures are collected, researched, and archived at the Braunschweig research institute. With the project DiASPora (Digital Approaches for the Synthesis of Poorly Accessible Biodiversity Information), a team led by Overmann was successful in the Leibniz Competition 2019 and is being funded with 1 million euros. This is where microbiology meets computer science in order to better exploit the enormous biodiversity of bacteria.
What was the motivation behind the foundation of the DiASPora project?
For several years we have been developing the database BacDive, which has become the largest meta database on microorganisms. Here we collect all existing data on taxonomy, cultivation, metabolism, origin, and molecular biology from various sources and offer them in over 600 data fields in a standardized format. Databases such as BacDive offer great potential for new findings, because - in contrast to data in the literature - they can be systematically evaluated. However, further-reaching, more complex analyses still require extensive bioinformatics knowledge and experience in the data sciences. For example, if the biochemical potential is to be determined from genome sequencing data and compared with metabolic data in BacDive, or if the physiology of microorganisms is to be derived from ecological data from other, scattered data sources. We want to lower these barriers and enable an improved understanding by means of state-of-the-art analyses, for example using artificial intelligence.
Which data should DiASPora connect and where does it come from?
We start with the data in our database BacDive, where we have a variety of data types. These we describe and link in a so-called knowledge graph. In addition, we extend these data with genome annotation data obtained from the numerous sequenced genomes of bacteria. The semantic processing then enables us to link these data with other data in the so-called Linked Open Data Network, where, for example, DBpedia/Wikidata also contains a lot of data from Wikipedia.
The data is to be semantically interlinked. What does that mean?
If you ask your smartphone today - whether it is Siri, Google Assistant or Alexa - "Name an Indian restaurant in my area that will be open tomorrow night", the algorithm can understand and answer this question. That it can answer the question is because the information on the Internet about Restaurants & Shopping is described in the Resource Description Framework language (RDF) and is therefore machine-interpretable (machine-readable). Although scientific data are much more complex, there have already been attempts to describe them in RDF format. In times of data flood, this kind of data preparation will become even more important in order to be able to fall back on the help of artificial intelligence when searching and linking data.
How can predictions about the properties of bacteria be made on this basis?
There are different ways to do this. The first is a purely phylogenetic or statistical one: If I know that all species in a taxonomic group have a property, for example that they form spores, then I can say with a certain probability that the next one I find in the group will also have this property. The second possibility is to use the genome information that is frequently available today. By systematically comparing genome information with phenotype information such as that from BacDive, I can successively improve the prediction of properties based on genome functions. Finally, the presence of the bacteria at a certain location - such as the occurrence in a hot, mineral hydrothermal spring - with simultaneous knowledge of the ecological conditions of this location also allows predictions about the physiology of the bacteria, specifically their growth at correspondingly high temperatures using inorganic compounds for energy production.
Do you focus on certain groups of bacteria and why?
Most microorganism species could not be described so far, because they are not yet cultivable in the laboratory. In order to make this huge "treasure" available for research, cultivation conditions must be determined empirically. Our idea is to make predictions specifically for this group and to test them in the laboratories of the DSMZ. One example is the acidobacteria that are dominant in the soil and are probably relevant for nutrient conversion. So far, only a very small part of the occurring species of these groups could be isolated and analyzed. Our new approaches should significantly improve our knowledge of this group, so that more novel bacterial strains can be isolated and made available for targeted studies.
What potential applications of this project do you see beyond basic research?
As already mentioned, RDF is already widely used in the commercial sector. If this could be significantly extended to the evaluation of scientific data, completely new analysis possibilities would emerge. If this trend continues, we will have better chances in the future to explore the gigantic flood of scientific data with semantic questions and to get answers and the underlying data much faster. The importance of the knowledge gained in this way could range from an improvement in the nutrient supply in agricultural soils, to the search for novel producers of active substances, to a better understanding of infectious agents.
Interview: Björn Lohmann