Protein-Design: Biomoleküle optimieren und entwerfen
Proteins are large biological molecules with a high molecular weight. The complex protein molecules are often referred to as molecular machines that keep the processes of life running. In cells and organisms, they perform a variety of tasks. For instance, they form cell structures (e.g. the cytoskeleton), act as accelerators of biochemical metabolic reactions (enzymes), transport substances (transport proteins) or recognize stimuli and pass them on (receptors and sensors). Proteins are also key players in energy storage, the immune system and gene regulation.
Proteins have a crucial role in numerous applications in science and industry: in biotechnology, for example, enzymes represent important specialized tools. Biotechnologically produced proteins are also used as medicines (such as antibodies or the peptide hormone insulin) and are important for numerous biochemical test procedures.
However, biotechnologists no longer rely solely on naturally occurring proteins. With the help of the latest findings and technologies, they can improve the function of existing proteins and tailor them for a specific range of applications. Protein engineering involves optimizing or redesigning proteins specifically for a task (explained below using the example of enzymes).
There are two different approaches:
-
Rational protein design: Based on data on the three-dimensional structure of an enzyme, obtained for example using X-ray crystallography, computer-aided predictions are made as to where the properties of the enzyme can be optimized. After modeling, the molecular blueprint of the enzyme is then specifically modified in the laboratory and the enzyme variants generated on this basis are tested experimentally.
- Directed evolution: This approach does not require three-dimensional structural data of the enzymes. Instead, an evolutionary process is simulated experimentally in the laboratory: Random mutations are triggered in the molecular blueprint, the corresponding gene of the enzyme. This results in a large number of enzyme variants. These variants are then tested in the laboratory in activity test series for improved properties (selection step). The best candidates undergo further optimization rounds.
Within a few months, enzymes can be adapted to new substrates or desired process conditions using these approaches. Combined with key technologies such as artificial intelligence (AI) and efficient high-throughput processes, development times can be shortened even further.
This in-depth report highlights how AI technologies have fundamentally changed structural biology and protein design in recent years.
Page 2 of 6
AI as a key technology
Artificial intelligence (AI) is a key technology of the 21st century that is transforming economies and societies worldwide. As a widely applicable tool, AI offers a wide range of opportunities. It is on its way to significantly change the way information and knowledge are handled in society, science and business. High-profile AI successes are primarily based on machine learning methods in conjunction with a significant increase in available and usable data as well as large and constantly growing computing power.
Since the US company OpenAI opened up access to its ChatGPT language model in November 2022, AI has been tested and used by millions of users for the first time. Since this milestone in AI development, generative AI has emerged as the most promising technology of our time.
Brief AI glossary
Artificial intelligence (AI) is a term for software and robotic systems that exhibit behavior that generally assumes human intelligence. At the same time, AI describes a branch of computer science that deals with the development of these systems.
Machine learning is a sub-area of AI. Computers learn to "recognize" patterns and regularities in a large amount of data through appropriate training.
Generative AI is a model of machine learning that learns from examples (training data) and can subsequently generate content.
Large language models (LLMs) are generative AI models that are trained with huge amounts of text. They are able to generate "natural-sounding" language by predicting which word is most likely to come next.
(Source: Plattform Lernende Systeme and Google Aufbruch Nr. 30)
The German government published an AI strategy in 2018, which was updated in 2023 with the BMBF's AI action plan. The BMBF alone is investing more than 1.6 billion euros in AI in the current legislative period with a focus on research, skills development, infrastructure development and transfer to application.
The future strategy for research and innovation adopted by the Federal Cabinet in February 2023 (Zukunftsstrategie Forschung und Innovation) also contains a number of references to AI. The aim is to actively shape transformation processes with the help of AI and safeguard Germany's technological sovereignty.
Page 3 of 6
Determining the shape of proteins
Proteins are long molecular chains consisting of amino acids as building blocks. As a rule, however, the amino acid chains are not thread-like, but twist and fold into complex three-dimensional structures.
The shape they take depends on the type and sequence of their amino acids - because these have different forces of attraction or repulsion. The interactions between the various amino acids of a protein are usually so complex that it has taken decades of research to understand the underlying principles.
The sequence of the amino acid building blocks of a protein - the so-called primary structure - can be deduced from the DNA sequence of a gene. However, the biological function of a protein depends crucially on the folding (secondary structure) and the resulting spatial shape (tertiary structure) and the type of interaction with other proteins (quaternary structure). It is the spatial structure that determines a protein's ability to interact with other proteins or cell components.
Experimental structural biology: data to feed the algorithms
Determining the structure of a protein experimentally is very time-consuming. In principle, this involves determining the individual atoms and their position in space. To do this, however, the highly dynamic protein - an enzyme, for example - must first be immobilized. Three methods in particular provide huge, highly complex data sets - which can only be analyzed with the help of computers.
X-ray crystallography examines molecules that must first crystallize. The structure of the crystal can then be calculated based on the diffraction of the radiation. Today, the process is a standard method in structural biology and has been used for around 90 % of experimentally determined protein structures.
In cryo-electron microscopy, an enzyme is frozen ultra-fast at -150 degrees Celsius so that it is no longer in motion. An electron micrograph is then produced.
Nuclear magnetic resonance spectroscopy is particularly interesting because it makes it possible to record enzymes in their momentum and possible different conformational states.
Page 4 of 6
Predicting protein structures with smart software
In general, the experimental methods used to determine the structure of proteins require expensive equipment, take a lot of time and are nonetheless fraught with uncertainties. Researchers in structural biology have long been looking for ways to theoretically deduce the molecular structure from the sequence of the individual amino acid building blocks.
However, a protein can consist of hundreds to thousands of amino acids. The astronomically high number of possible combinations of amino acids and their interactions with each other makes research into the 3D structure a huge puzzle. The process is very time-consuming, even with modern computer technology. However, calculating how a protein will fold based on its primary structure requires enormous computing capacity. The idea is that the laws of chemistry only allow certain options and a protein will probably adopt the folding that is the most energetically favorable from these options.
Protein folding with combined computing power
The Folding@Home project, developed by a team at Standford University, was launched in 2000. Here, thousands of private computers are networked via the Internet, which make their resources available for the research tool whenever they are idle. As a citizen science project, Folding@Home is primarily looking for the causes of protein misfolding that are associated with diseases such as Alzheimer's or Huntington's disease. The basis for this is formed by so-called Markov models, which use probability theory to model randomly changing systems.
Rosetta@Home, which was launched in 2005 by David Baker's team at the University of Washington in Seattle, is based on a similar principle to Folding@Home. It was developed on the basis of the software BOINC. Rosetta divides a protein into short sections of amino acid sequences for which there are similar sequences in other proteins for which the corresponding spatial structure is known. The software gradually assembles the overall structure from the individual structures determined in this way. The Baker Lab's aim with Rosetta is not only to frequently arrive at an accurate result, but also to make precise structural predictions. Only then can the tool be used to design new proteins.
Around 44,000 computers are currently making their free computing power available to Rosetta and, similar to Folding@Home, the COVID-19 pandemic has provided a performance boost. Until 2020, Rosetta was considered the gold standard for predicting protein structures. Nevertheless, the software only came moderately close to experimentally determined structures in terms of the accuracy of its predictions.
AI revolution in protein folding research
Artificial intelligence, with its particular strength in pattern recognition, has ushered in a new era in protein folding research. Machine learning algorithms are getting better and better at predicting how a protein will fold three-dimensionally into a ball based on its amino acid sequence.
The publication of AlphaFold, a deep learning algorithm from the British company Deepmind, was groundbreaking for protein research in 2020. The AI was trained with all the protein data from the Protein Data Bank (PDB) which had been determined experimentally up to that point. To do this, the developers fed the algorithm with the amino acid sequence of 170,000 proteins and their corresponding spatial structure. From this, the software derived patterns and correlations that it uses to predict the 3D structure of unknown sequences.
The performance of the software was impressively demonstrated in the scientific competition for computer-aided structural biology CASP (Critical Assessment of Structure Prediction). The aim of CASP is for bioinformaticians around the world to submit their computer-aided structure predictions for the spatial shape of biological molecules for which experimental structural data is already available but has not yet been published.
AlphaFold in 2018 and especially its successor AlphaFold2 in 2020 left all other participating teams at CASP far behind. They were able to predict with a high degree of reliability how an amino acid sequence would fold. The journal "Science" named AlphaFold the scientific breakthrough of the year 2021. Deepmind is now part of the Alphabet Group, which has made AlphaFold2 available to the general public under an open source license.
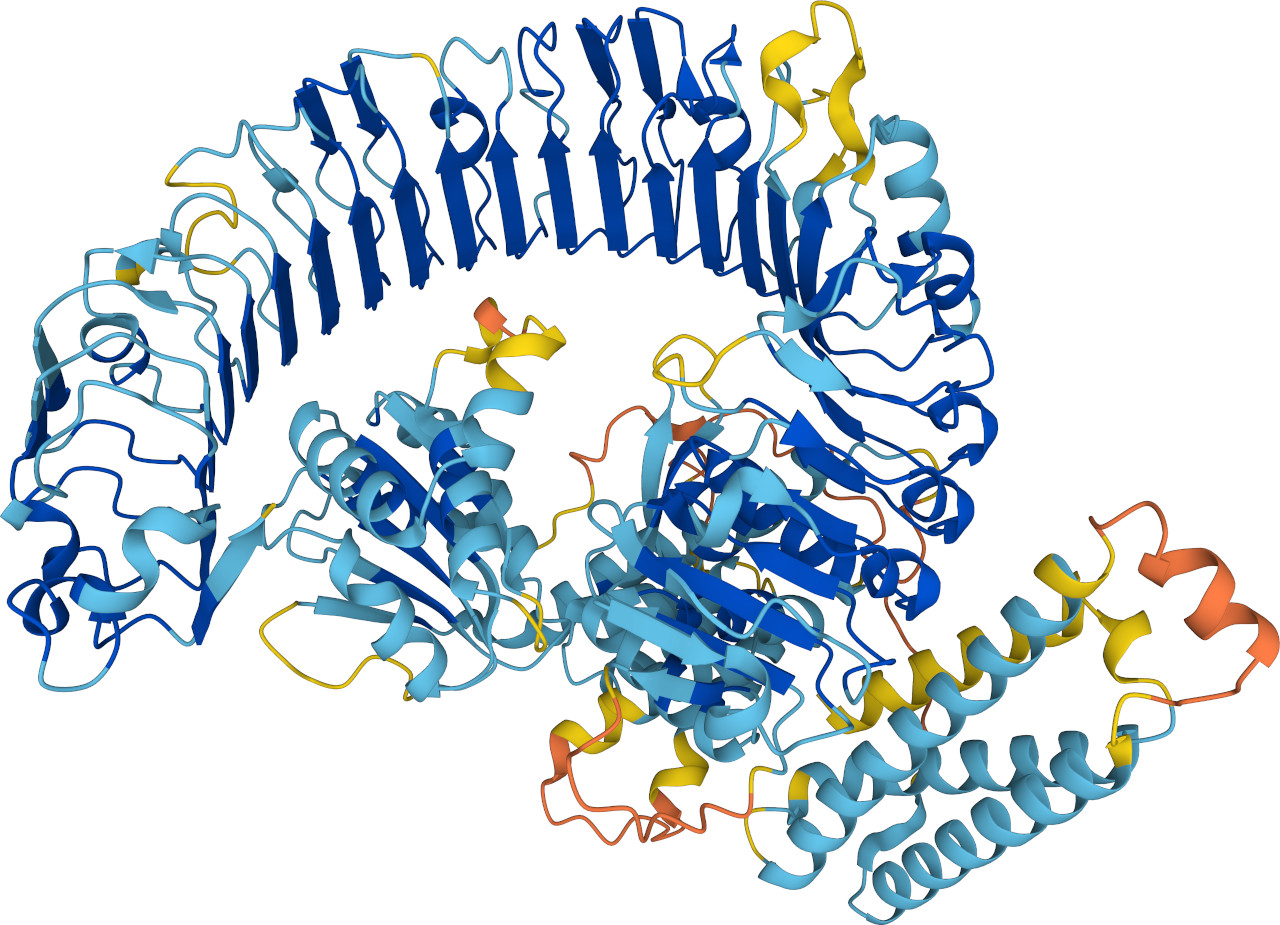
200 million protein structures simulated
Together with the European Bioinformatics Institute (EMBL-EBI), the DeepMind team has built up the AlphaFold protein structure database.
Among other things, AlphaFold2 was used to determine the structure of the proteins of the COVID-19 pathogen SARS-CoV-2. This made it possible to produce vaccines and medicines. Since July 28, 2022, the database has included structure predictions for around 200 million protein models.
The Baker Lab followed suit in 2021 and introduced RoseTTAFold, a software that achieves a similar level of precision to AlphaFold2.
Nevertheless, AlphaFold2 has some weaknesses. For a long time, it was only able to predict monomers, but not protein complexes. Nor does the algorithm take cofactors into account. This task is now set to be handled by a separate model called AlphaFill. Additionally, the model partly relies on the premise that similar proteins share a similar evolution. Concerning predictions for synthetic proteins, where this co-evolution doesn't exist, this could lead to errors. Lastly, AlphaFold2 always determines only one structure for a protein, although proteins can indeed exist in multiple folds.
For example, while AlphaFold2 gives an idea of the structure of a protein, X-rays and high-resolution cryo-electron microscopy usually still provide the more precise structures needed to understand enzymatic reactions or develop drugs. Nevertheless, AlphaFold2 and RoseTTaFold have quickly become an integral part of the work of structural biologists and enzyme designers worldwide and have transformed protein research.
Strengths and weaknesses of AlphaFold2 at a glance
What AlphaFold2 can predict: Folding of individual protein chains, protein multimers, protein-protein complexes with multiple subunits
Where AlphaFold2 struggles in predictions: Different conformations of the same amino acid sequence; effects of point mutations; antigen-antibody interactions
What AlphaFold cannot predict: Protein-DNA and protein-RNA complexes, nucleic acid structures, ligand-ion binding, post-translational modifications; the adjacent membrane layer in transmembrane domains of proteins
Source: EMBL-EBI Training
Page 5 of 6
Protein structure predictions with large language models
The release of AlphaFold2 in July 2021 was groundbreaking for structural biology. In November 2022, the "ChatGPT moment" followed: the company OpenAI published the large language model GPT and the chatbot based on it, catapulting the topic of generative AI into the mainstream.
GPT stands for Generative Pre-Trained Transformer. The model is based on a neural network architecture that resembles the structure of the human brain and is therefore called Transformer Architecture.
Experts casually refer to the large language models as stochastic parrots. The principle is surprisingly simple: GPT-4, the current 2023 form, has - to put it simply - done a lot of reading. Wikipedia entries, the Gutenberg project, books, letters and more. On this basis, the chatbot determines, letter by letter, what the most likely answer to a question or task is. During the training phase, the program then received feedback on its answers from humans in order to improve. Because ChatGPT strings together letter after letter purely stochastically, it has no understanding of the content of its answers. Where it cannot find answers, it "hallucinates" invented answers and sources. Even so, ChatGPT achieves unprecedented functionality.
Language models for protein structure prediction
In 2022, the Facebook parent company Meta presented ESMFold, a large language model for protein structure prediction, based on the same principle as GPT. Instead of building sentences stochastically from the letters of the alphabet, ESMFold uses the amino acids as letters and assembles proteins from them. In order to learn the "language of proteins", the program first learned to fill in "gap texts", i.e. to correctly fill in omissions in amino acid sequences. In this way, the AI developed a sort of intuitive understanding of protein sequences.
In a second step, the AI then combines this understanding - similar to AlphaFold2 - with the knowledge of the interrelationships between sequences and structures that comes from experimentally determined protein structures, but also from structures predicted by AlphaFold2.
As of 2023, ESMFold is slightly less precise than AlphaFold2. However, the algorithm is 60 times faster, especially for shorter sequences with up to 1024 amino acids. Within two weeks, ESMFold made structure predictions for 617 million proteins. The data examined was so-called metagenomic DNA from environmental samples - a large mix of the genetic material of countless microorganisms, most of which have never been cultivated. The data is available in the ESM Metagenomic Atlas and now totals 772 million proteins.
Around a third of these predictions are considered to be so good that they are often correct down to the atomic detail. However, millions of structures are completely different from what is known from research to date, including the structural predictions of AlphaFold2. This suggests that the world of proteins within the still unexplored microorganisms is far more diverse than what has been studied in the world's laboratories to date.
The many millions of structures to which ESMFold itself assigns a low reliability point in a similar direction: Could it be that numerous proteins do not have one defined structure, but are highly dynamic? In any case, many experts assume that such an AI model is particularly well-suited to predicting how a protein changes when individual amino acids are replaced.
So far, the expectation that language models can predict proteins for which no related molecules are known more effectively than AlphaFold2 and RoseTTAFold has not been fulfilled. After all, the latter rely heavily on sequence analogies. In the most recent CASP competition, ESMFold did not prove to be superior in this respect.
Page 6 of 6
Using AI models to create novel designer proteins
Understanding protein structures and optimizing enzymes so that they work more specifically or with higher turnover rates is just as important in medicine as it is in industrial biotechnology. Synthetic biology refers to approaches for the targeted creation of biological systems with novel properties. This also includes the production of artificial proteins that are optimally designed for a specific purpose. For a long time, trial and error played an important role when researchers combined structures of sections of known proteins. Nowadays, this field of research is also dominated by AI technologies.
Language models for protein design
Numerous protein language models have been created since 2022. For numerous classes of proteins, structures can now be determined or generated on computers with the same accuracy as through experimental methods. However, "intrinsically disordered proteins", i.e. flexible molecules without a fixed structure, proteins with cofactors and large protein complexes, still pose difficulties. On the other hand, experts see opportunities in novel sequences that deviate greatly from known structures and could still be functional.
The David Baker Lab, for example, has developed RFdiffusion to create proteins that evolution has never produced. Although the software is based on a protein language model and therefore works stochastically, it appears to have a better command of many of the rules of protein folding than its creators.
A team led by Burkhard Rost from the Technical University of Munich has developed the protein language model EMBER2. Here, too, the researchers focused on ensuring that the software works independently of sequence analogies. As a result, the model does not quite achieve the quality of AlphaFold2. However, EMBER2 is particularly good at predicting unusual protein structures and can also design novel proteins - at a lower cost than the competition from Alphabet.
There are now numerous language models that are also used for protein design - including ProGen, Chroma and ProtGPT2.
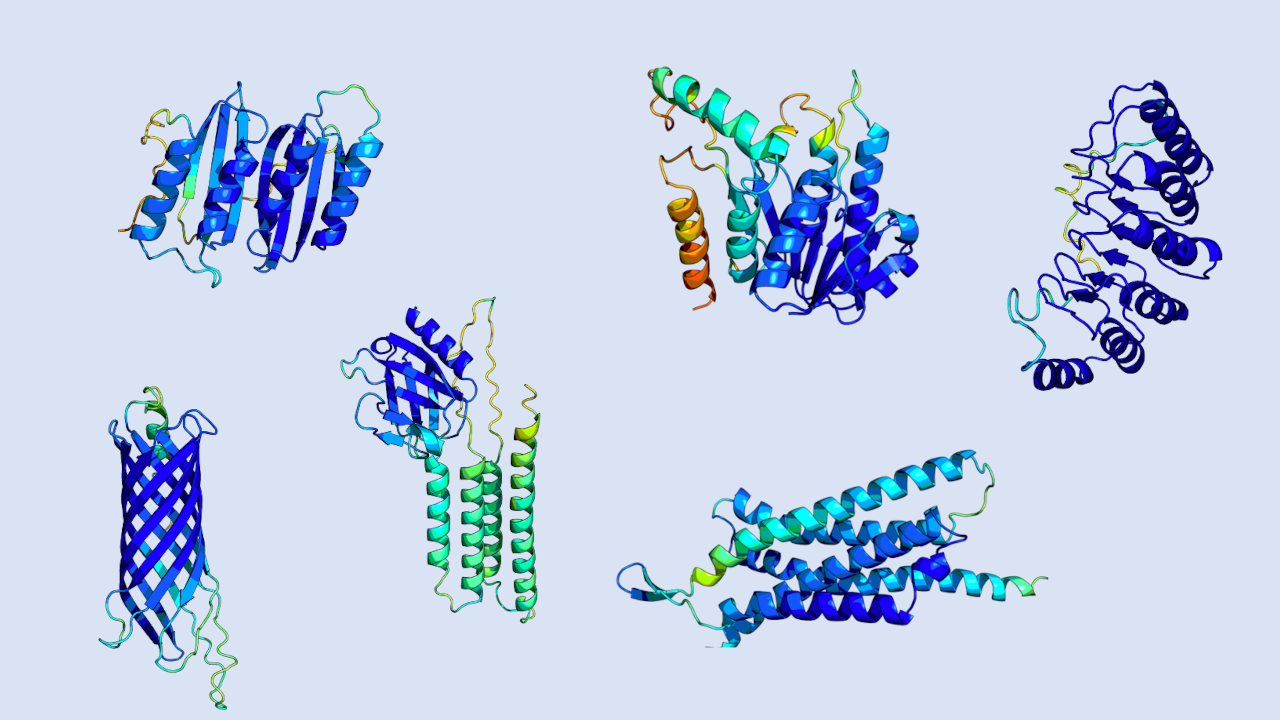
Creating proteins with ProtGPT2
ProtGPT2 was developed by Birte Höcker's team at the University of Bayreuth. Her team trained the language processing model with 50 million sequences of natural proteins. "Now it not only understands the language of proteins, but can also use it creatively," says Birte Höcker in an interview with bioökonomie.de.
It can be used to design proteins that adopt stable structures through folding and are permanently functional in this state, says Höcker. Uniquely, ProtGPT2 generates proteins that have such an intrinsically differentiated structure that they are already operational in their respective environment. "We also have evidence that the model can create proteins that do not occur in nature and may never have existed in the history of evolution," says Höcker. "This opens the door to innovative research that creates previously unknown proteins."
Not all AI tools are generally accessible
One particular concern for academic researchers and start-ups is that seven of the nine transformers currently used for proteins were developed by large corporations. This is mainly due to the enormous costs and the computing power required to train the AI. Nevertheless, some companies have made their products freely available, such as AlphaFold2 and ProtTrans.
As promising as the success of language models in designing new proteins has been so far, there is still a lot to improve. The so-called attention models, which enable most language models to focus on relevant parts of the input data and use them specifically for processing, are not designed for three-dimensional structures. The majority of models work most reliably within conventional protein structures, limiting the potential for innovation.
And last but not least, large AI models have high energy requirements and therefore a very high carbon footprint. Researchers are working on more data-efficient and energy-efficient models.
Opening up new avenues in protein research
However, even though there are still some challenges to overcome, there is largely consensus within the research community that work on protein structures is no longer conceivable without AI. In addition, the success rates of protein design have increased enormously thanks to technological advances, says Birte Höcker. "In the past, many designs failed at the production stage - for example, due to protein expression in bacterial cells. Today, we are seeing a significant improvement in the properties and handling of many designer proteins, allowing us to ask completely new questions and tackle entirely new challenges."